21
Jul
Generative AI on the edge – Why and what is needed
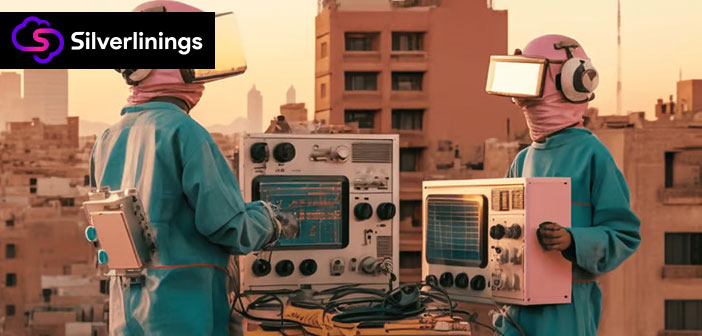
In the fast-moving generative AI (Gen AI) market, two sets of recent announcements, although unrelated, portend why and how this nascent technology could evolve. The first set was Microsoft’s Office 365 Copilot and Adobe’s Firefly announcements, and the second was from Qualcomm, Intel, Google and Meta regarding running Gen AI models on edge devices. This evolution from cloud-based to Edge Gen AI is not only desirable but also needed, for many reasons, including privacy, security, hyper-personalization, accuracy, cost, energy efficiency, and more, as outlined in my earlier article.
While the commercialization of today’s cloud-based Gen AI is in full swing, there are efforts underway to optimize the models built for power-guzzling GPU server farms to run on power-sipping edge devices with efficient mobile GPUs, Neural and Tensor processors (NPU and TPU). The early results are very encouraging and exciting.
Gen AI Extending to the Edge
Office 365 Copilot is an excellent application of Gen AI for productivity use cases. It will make creating attractive PowerPoint presentations, analyzing and understanding massive Excel spreadsheets, and writing compelling Word documents a breeze, even for novices. Similarly, Adobe’s Firefly creates eye-catching images by simply typing what you need. As evident, both of these will run on Microsoft’s and Adobe’s clouds, respectively.
These tools are part of their incredibly popular suites with hundreds of millions of users. That means when these are commercially launched and customer adaption scales up, both companies will have to ramp up their cloud infrastructure significantly. Running Gen AI workload is extremely processor, memory, and power intensive—almost 10x more than regular cloud workloads. This will not only increase capex and opex for these companies but also significantly expand their carbon footprint.
One potent option to mitigate the challenge is to offload some of that compute to edge devices such as PCs, laptops, tablets, smartphones, etc. For example, run the compute-intensive “learning” algorithms in the cloud, and offload “inference” to edge devices when feasible. The other major benefits of running inference on edge are that it will address privacy, security, and specificity concerns and can offer hyper-personalization, as explained in my previous article.
This offloading or distribution could take many forms, ranging from sharing inference workload between the cloud and edge to fully running it on the device. Sharing workload could be complex as there is no standardized architecture exists today.
What is needed to run Gen AI on the edge?
Running inference on the edge is easier said than done. One positive thing going for this approach is that today’s edge devices, be it smartphones or laptops, are powerful and highly power efficient, offering a far better performance-per-watt metric. They also have strong AI capabilities with integrated GPUs, NPUs, or TPUs. There is also a strong roadmap for these processor blocks.
Gen AI models come in different types with varying capabilities, including what kind of input they utilize and what they generate. One key factor that decides the complexity of the model and the processing power needed to run it is the number of parameters it uses. As shown in the figure below, the model size ranges from a few million to more than a trillion.
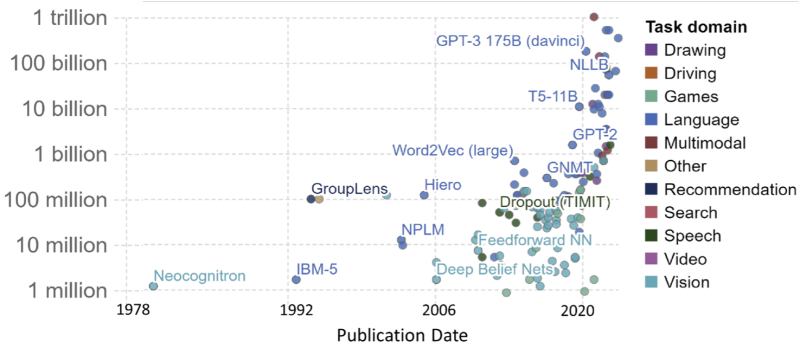
1 comments